
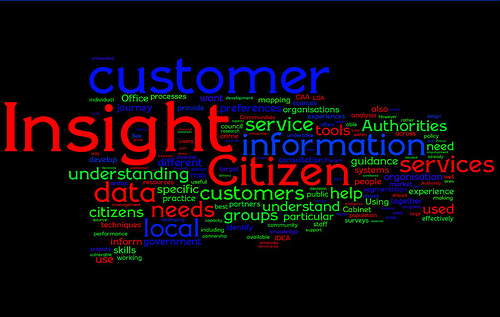
Statistical significance indicates that the analysis results may be interpreted as being a reliable estimator of the “real” effect that the campaign had on its target audience.įor each of the proportion and T-test statistical tests, three factors determine whether the results were statistically significant (i.e., whether they have a p-value = 0.05): When a campaign is deemed statistically significant, it implies that the campaign results were most probably not due to chance. T-test: This test determines whether the average per-customer metric results observed are statistically different between the test and control groups (e.g., did test group customers exhibit higher average spend amounts as compared with the customers in the control group?).įor each of the two tests a p-value should be calculated, and both p-values should then be used to derive the tests’ statistical significance (p-value = 0.05 is used to indicate significance).Proportion test: This test compares the average response rates of the test and control groups, i.e., the percentage of each group’s customers who performed some tracked action during the campaign measurement period.There are various techniques for measuring campaign effectiveness in terms of uplift and statistical significance. Calculating Statistical Significance for Customer Marketing Campaigns In order to determine how likely the calculated uplift was, in fact, a direct result of the campaign, the statistical significance of the result must be calculated.

However, the resulting uplift calculation may or may not be a reliable indicator of the impact of the campaign itself. The goal is to understand how much impact the campaign had on any particular uplift metric (such as an increase in the amount that customers spent), by analyzing the differences in behavior between the test and control group. The most reliable way to measure campaign effectiveness is to split the campaign’s target audience into two separate groups and to compare the resulting behavior of each one: a test group (those customers that actually receive the campaign) and a control group (customers similar to those in the test group, but who received no campaigns during the campaign measurement period). Calculating Uplift using Test and Control Groups Instead, the marketer should engage in additional experimentation (for example, by making changes to the campaign or fine-tuning the recipient groups) with the goal of achieving satisfactory uplift results and statistical significance. However, in the event that an uplift result is deemed to be not statistically significant, then the marketer should not rely on that uplift result for decision-making. When a campaign’s calculated uplift is determined to be statistically significant, there exists strong evidence that the campaign was responsible for the increase in spend (or any other uplift metric analyzed). Stated another way, statistical significance in campaign analysis is the parameter which indicates whether the campaign recipients’ behavior was the direct result of a specific campaign, or whether similar results might have been observed even had the campaign never been run. When analyzing the results of marketing campaigns, statistical significance is a probabilistic indication of whether or not the observed campaign results would have likely occurred even in absence of the campaign.
